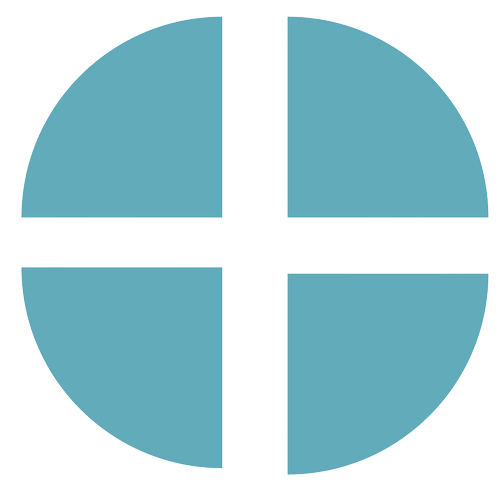
AI in Practice Part 1: Proprietary Data and Compliance
How do you ensure compliance and protect your customers’ data while using an LLM?
.png)

This is Part 1 of the AI in Practice Series.
Part 1: Proprietary Data & Compliance
Part 2: Product Strategy
Part 3: Organizational Change
We are in the implementation phase of AI technology. While hype is fun, we want to help executives grapple with the big question: what the hell do you do now? How does AI change the product roadmap? What about company culture? Pricing? Data privacy?
In short, we need to move from hype-driven blog posts to pragmatic implementation guides. These topics are especially important because so many of the companies we’ve talked with are struggling to deploy generative AI projects at scale. According to an S&P Global study, nearly 70% of respondents have at least one AI project in production but less than half of those (28% of respondents) have reached enterprise scale. In addition, 31% of respondents are still in the pilot or proof-of-concept stage.
To figure it out, we put together two round table sessions with 25+ different executives: CEOs, CTOs, CPOs, and AI leads from 21 different companies across a variety of industries. The group represented a mix of 17 private companies, with an aggregate of $30B+ in valuation and $5B+ in funding, and 4 public companies.
We’ve compiled, anonymized, and written down the best insights from those working sessions. The takeaways are diverse and enlightening. We are entering into a new age of software, and many previous best practices will have to be reconsidered. We’re publishing a series that will cover a variety of topics across data privacy and compliance, product strategy, pricing, resourcing, UI/UX, and culture.
Today, we want to cover the most pressing issue: proprietary data and data privacy/compliance. The top question on the minds of every executive we spoke with was about data privacy and compliance. How do you ensure compliance, and protect your and your customers’ data?
“Do you find that they’re unaware of where their data is going? Or are they just basically saying, yeah, that’s fine? Because I think there are camps that are unaware, there are camps that are really concerned, and then there are also these tools that are early in a lot of ways. We’re just trusting them that they’re not going to use that data, and there’s not really a long track record of responsibility around it. Which yields out wanting to self-host that, or control the instances, so you can put your data back in control of your hands.” - CTO at $1B+ Private Tech Company
There is a wide spectrum of concerns around data, and many strategies have emerged for protecting proprietary data and ensuring data privacy and compliance.
Managing for Proprietary Data
For some companies, proprietary datasets are a major part of their competitive advantage. For others, it is important but less critical to defensibility. Regardless, most businesses are loath to share data with others. The rise of LLMs and generative AI has brought to the foreground questions around ownership and what is and isn’t proprietary (exhibit A: the numerous LLM lawsuits afoot today and 20% of the top 1000 websites blocking AI crawler bots). Those few companies that haven’t sued an AI provider are grappling with how to best manage and protect their data from LLMs. The executives we spoke with shared a few strategies:
Trust the LLM providers
Decisions about foundation models mirror those around cloud hosting. Deciding what foundation model to use will be similar to choosing your cloud vendor. At a baseline, double check there are clauses in the contract to ensure data isn’t being used for training. Many companies at this point choose to trust the “do not train” clause in the OpenAI agreement—infringing on this agreement would be a company-ending event for OpenAI.
Ultimately, the level of trust required is the same as your cloud vendor (you process your data in their data pipelines and trust they don’t take it or share it). Select the right tool or platform that has protected APIs and tools you can leverage for fine-tuning models with your own data.
“Legally, you’re protected. Do you really trust them? That’s going to be a judgment call.” - VP of Product at $100B+ Public Tech Company
Refrain from sharing proprietary data
The proprietary data you own doesn’t have to be in the model. There are protective layers you can explore that will allow you to combine your data and the user prompt to generate a more custom response from the LLM—tools like embedding APIs or fine tuning APIs. Embedding APIs transform your data into abstract representations that the LLM understands and can use to generate more specific responses, without giving away the actual content. Fine tuning APIs refine an LLM’s understanding to be aligned with specific industry or domain nuances while preserving data confidentiality. These tools allow you to put in proprietary content and get insightful data back without sharing that content.
“One thing I’ve learned about actually getting into the APIs and the docs that are available for these tools [...] is you don’t need your proprietary data to be in the model. The model, like GPT-4, was teaching computers to speak human. Then they have additional APIs that you layer on top of that, that let you insert your proprietary data to let the LLM talk about your subject matter. [...] That’s the brilliance of what OpenAI built and the way they designed their APIs. They did the hard work to make the computer speak English – or any language – and then you can layer in your proprietary content, along with the user's prompt, and get really insightful content back. That’s how we’ve been using it with our support documentation.” - CEO at $500M+ Private Tech Company
Train your teams on data issues.
Data sharing is a concern if you paste things into a public interface. There is a different type of education around this type of data privacy. Guardrails need to be put in place for internal use cases. Otherwise, there could be a free-for-all where people take data and put it into places where they shouldn’t.
“You have to train your teams about how they’re using the different versions, of course, to make sure your data is protected. We put out general education with our team around that data privacy. Basically, it’s a simple answer for us. We just say, ‘If you wouldn’t post a blog post about it publicly, then don’t put it into the ChatGPT model publicly.’” - Co-founder at $1B+ Private Tech Company
Managing for Compliance
The other crucial concern for many executives is ensuring compliance. Data privacy concerns have grown substantially over the past decade, and failure to comply can result in hefty fines (see Meta’s $1.3B fine) that could be business-ending. The strategies around proprietary data and data compliance are crucial for businesses looking to invest in AI. Here are some things to keep in mind to avoid costly consequences related to subpar AI data management.
Start with where the data is coming from
Data provenance is a key area to invest in before doubling down on AI. You need to make sure that the data you’re training on includes the metadata that describes where that data came from. Who? When? Where? Having the answers to these questions will protect you against legal issues down the line.
Customer data leakage
Protect the prompts sent to OpenAI and the potential customer data that could be shared. Ask yourself: “Do I have the right to send customer data?” This involves figuring out what is embedded within your legal terminology. Your customers want to be reassured that their data is protected and will not be shared with others. Hard rails and consistent monitoring of data usage will ensure there is no data exfiltration.
Keep an eye on regulation
Many industry members are advocating for regulation, and it is important to continuously monitor the ongoing developments. While there has been a rush for technical AI talent, there is a compelling need for a good AI lawyer for those who are serious about AI. Things change quickly, this is already very complicated, and there will almost certainly be big fines with tough provisions.
Open-source alternatives are to-be-assessed
Open source could eventually be a solution to all of this, but it’s not the right answer for many at this point—as we will discuss in our next release.
If you have feedback or want to discuss strategies related to building with LLMs, you can reach us at knowledge@tidemarkcap.com. To learn more about how we think about AI as an opportunity and a threat, visit our essay here. If you’d like to keep up as we dig deeper into AI implementation strategies and get notified when we release our next essay on product strategy, make sure to subscribe below.
AI in Practice Series
Part 1: Proprietary Data & Compliance
Part 2: Product Strategy
Part 3: Organizational Change
Stay in the Loop
Enter your details and be notified when we publish new articles on The Highpoint.
